Gen Z + QSR: The Inside Scoop On the Next Generation of Consumers
We surveyed Canadian and American Gen Z to understand their fast-food ordering habits & routines and identify key opportunities for brands.
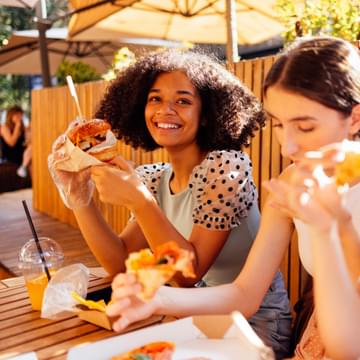
Imagine - a newsletter that's genuinely useful.
Get free data-driven reports, interviews with business legends, and monthly tips on research best practice.